BIOS IT Blog
AI & Supermicro: The Engines Driving a New Era for Healthcare
Posted on 26 September, 2022
Introduction
One of the sectors that will see huge benefits of the 4 th industrial revolution is the healthcare and life sciences field. In fact, Gartner predicts that AI and 5G technologies are so profound, that 75% of organisations will move from piloting AI to operationalising it.
AI offers incredible potential in terms of cutting healthcare costs, improving treatment, and bolstering accessibility. Accenture predicted that the top AI applications may result in annual savings of $150 billion by 2026.
Not only could AI solve the problem of a lack of qualified clinical staff by taking over some of the diagnostic duties typically allocated to people, but it also offers dramatic advantages over traditional analytics and clinical decision-making techniques. Learning algorithms can become more precise and accurate as they interact with training data, allowing healthcare professionals to gain extraordinary insights into diagnostics, care processes, treatment unpredictability, and patient outcomes. It will help with human learning too, and it can take people through naturalistic simulations in a way that simple computer-driven algorithms cannot.
According to the American Cancer Society, the accuracy levels of mammograms cause 1 in 2 healthy women to be told they have cancer, but the use of AI is enabling review and translation of mammograms 30 times faster and with 99% accuracy. It is also already helping professionals to better define the aggressiveness of cancers and target treatments, and may enable the next generation of radiology tools, perhaps accurate enough to replace tissue samples altogether.
Pattern recognition is also starting to be used to identify patients at risk of developing a condition – or identify the way in which it can deteriorate due to lifestyle, environmental, genomic, or other factors. It can also help clinicians to take a more comprehensive approach and better coordinate long-term treatment programs.
What’s more, according to the California Biomedical Research Association, it takes an average of 12 years for a drug to travel from the research lab to the patient. Only five in 5,000 of the drugs that begin preclinical testing ever make it to human testing and just one of these five is ever approved for human usage. It costs a company on average US $359 million to develop a new drug from the research lab to the patient – but AI has the potential to significantly cut both the costs and the time to market for new drugs.
AI could even revolutionise end of life care, and help people to remain independent for longer, thereby reducing the need for long-term hospitalisation and care homes.
Implementing AI into specific areas of healthcare has already shown remarkable potential in:
Radiology
- A deep-learning-based algorithm was developed using 50,000 normal chest images and 7,000 active TB scans.
- Outperformed radiologists during tests
Dermatology
- Deep-learning neural-network trained by feeding it 100,000 images of malignant melanomas and benign moles.
- Its performance was compared with 58 international dermatologists, who had an accuracy of over 86% while the neural network detected 95%.
Oncology
- AI can be used in the future to understand cancer more and treat it.
- This field is very promising, but has not seen too much real-life application, rather it has been used to make better judgments.
Cardiology
- Heart disease related complications are the most prevalent cause of death. Faster AI based predictions will result in earlier detections prompting preventative actions.
- Cardiovascular diseases often manifest in the eye – AIs are being taught to notice risk factors like age, gender, smoking status, blood pressure, etc.
The better the model, the better the AI. Larger models require more digital data. We have that in increasing abundance with each passing day. In fact, 90% of the world’s data has been gathered in the past two years.
Managing such large quantities of data requires large amounts of computing power. Thankfully, that power is finally in our hands. With the increase of readily available data, AI model sizes grew from tens-of-millions of parameters in 2017 to hundreds-of-billions in 2020 – and these model parameters double around every two and a half months. If this trend continues, the number of parameters is set to reach trillions by the end of 2023.
Supermicro has been working on industry consortiums to build a deep distributing learning model for healthcare. A focal point of this solution is that raw data is not shared between systems, hospitals, or other parties, to help maintain the integrity of the industry – so, only the models will be shared. This way, all inferencing and training happens in real-time.
This was achieved by making use of open-source frameworks, such as MONAI, which distributes the models, NVIDIA CLARA, a healthcare application AI powered imaging, genomics, and development and deployment of smart sensors, and NVIDIA FLARE, another open-source framework (FLARE stands for Federated Learning Application Runtime Environment, which underlies the aforementioned CLARA. This entire architecture is held together by Kubernetes and CEPH – storage management software that cover each other’s shortcomings).
Regardless of the implementation, the common feature is that more than one system will be working together for one task. In the case of healthcare, that means sensors, inferencing systems, data transfer between systems, local storage, and training systems, to name a few. These separate systems will make use of the building-block technology, with each having classified and defined verticals.
The first vertical is cloud technology, which is at the core when using OpenStack or vCenter, for example. The second vertical is storage: sensors, inferencing, training, etc., all need storage at some point or another – including high-performance storage, scalable or archiving storage, for example. The third (and fastest-growing) vertical is Artificial Intelligence/ Machine Learning (AI/ML), and other emerging technologies, which requires different stacks of software, tools, and environments, as well as a dedicated team to manage and provide solutions. The fourth is the Internet of Things (IoT), which includes any device that picks up and provides new information, such as sensors and scanning devices. The fifth and final vertical relates to the enterprise applications that govern the financial, CMDB, and accounting systems for all the data used.
This infrastructure is made possible by tight-knit collaboration with other companies that specialise in certain parts of the process. Supermicro handles two types of requests: POC and small deployments – whereby Supermicro and their partners pre-design and pre-validate solutions for quick and easy fixes or upgrades, and requests from Fortune 100 companies. The main difference between the two types is the scale of deployment and purpose of use. Because of the enormous scale use of the system, it experiences much more strain, resulting in a more rigid benchmarking being performed. In terms of deployment, Supermicro acts as a liaison between re-sellers and the investing party.
Working with such large amounts of data and parameters means that theoretically, any system can run software, but the time it takes to get a result will vary drastically. There are heavy-duty IT setups that are capable of crunching terabytes of data to reach an accurate diagnosis from days to mere hours.
And when human lives are at stake, every second counts.
Not what you're looking for? Check out our archives for more content
Blog Archive
From Silicon to Cloud
Turnkey IT Solutions that scale to meet customers needs
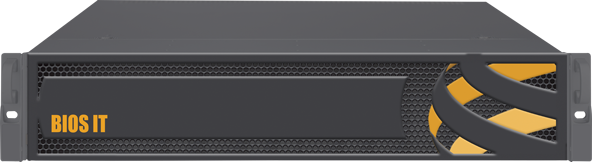