BIOS IT Blog
NVIDIA® DGX-2™ in the Cloud ResNet Results
BIOS IT's Cloud partners vScaler recently integrated the NVIDIA DGX-2 monster server into its cloud environment and subsequently put it to the test.
What is ResNet?
ResNet (short for Residual Network) has been hailed as groundbreaking in the world of AI and Deep Learning, making it possible to train up to hundreds or even thousands of Neural Network layers and still maintain compelling performance.
AI breakthroughs and advancements are dependant on deep convolutional neural networks. In theory, the deeper the model is, the more complex the task that can be solved. However, the training of the neural network also becomes more difficult and the accuracy can start to saturate and degrade. ResNet tries to solve these challenges with training Deep Neural Networks and as such is used as a baseline for assessing training and inference performance.
The NVIDIA DGX-2 Server
Increasingly complex AI demands unprecedented levels of compute. NVIDIA DGX-2 is the world’s first 2 petaFLOPS system, packing the power of 16 of the world’s most advanced GPUs providing a quantum leap in accelerating the newest deep learning models over traditional CPU architectures.
Introducing vScaler
vScalerTM is a cloud platform that enables anyone to quickly deploy scalable, production-ready deep learning environments via an optimised private cloud appliance. Users can spin up application-specific software stacks with the appropriate Deep Learning frameworks installed and ready for use, including Tensorflow, Caffe and Theano. These frameworks are accelerated using the world’s fastest GPUs, purpose-built to dramatically reduce training time for Deep learning and Machine Learning algorithms and AI simulations.
With the recent integration of the NVIDIA DGX-2 server, BIOS IT, on behalf of vScaler, can now offer users remote access to this monster machine within the vScaler cloud environment.
The Results
Comparing the average images classified per second for various models with a fixed batch size and varying GPU count shows the near linear performance increase for each GPU added. For example, when running ResNet-50 with a batch size of 256, going from 1 GPU to 16 GPUs results in a scaling factor of 13.9 (which represents an 86% efficiency in scaling) and an astounding figure of over 12,000 images classified per second. The tech team at vScaler are confident that they can still improve this further with some more optimizations. In comparison with legacy x86 architectures, DGX-2’s ability to train ResNet-50 would require the equivalent of 300 servers with dual Intel Xeon Gold CPUs costing over $2.7 million dollars.
See the full benchmark results here.
Get in touch with us today to arrange remote access to the worlds most powerful Deep Learning Solution - DGX-2 in the Cloud!
Not what you're looking for? Check out our archives for more content
Blog Archive
From Silicon to Cloud
Turnkey IT Solutions that scale to meet customers needs
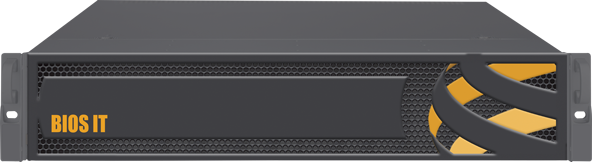